In information retrieval, tf–idf or TFIDF, short for term frequency–inverse document frequency, is a numerical statistic that is intended to reflect how important a word is to a document in a collection or corpus.[1] It is often used as a weighting factor in searches of information retrieval, text mining, and user modeling. The tf–idf value increases proportionally to the number of times a word appears in the document and is offset by the number of documents in the corpus that contain the word, which helps to adjust for the fact that some words appear more frequently in general. Tf–idf is one of the most popular term-weighting schemes today; 83% of text-based recommender systems in digital libraries use tf–idf.[2]
Variations of the tf–idf weighting scheme are often used by search engines as a central tool in scoring and ranking a document’s relevance given a user query. tf–idf can be successfully used for stop-words filtering in various subject fields, including text summarization and classification.
One of the simplest ranking functions is computed by summing the tf–idf for each query term; many more sophisticated ranking functions are variants of this simple model.
Term frequency
Suppose we have a set of English text documents and wish to rank which document is most relevant to the query, “the brown cow”. A simple way to start out is by eliminating documents that do not contain all three words “the”, “brown”, and “cow”, but this still leaves many documents. To further distinguish them, we might count the number of times each term occurs in each document; the number of times a term occurs in a document is called its term frequency. However, in the case where the length of documents varies greatly, adjustments are often made (see definition below). The first form of term weighting is due to Hans Peter Luhn (1957) which may be summarized as:
- The weight of a term that occurs in a document is simply proportional to the term frequency.[3]
Inverse document frequency
Because the term “the” is so common, term frequency will tend to incorrectly emphasize documents which happen to use the word “the” more frequently, without giving enough weight to the more meaningful terms “brown” and “cow”. The term “the” is not a good keyword to distinguish relevant and non-relevant documents and terms, unlike the less-common words “brown” and “cow”. Hence an inverse document frequency factor is incorporated which diminishes the weight of terms that occur very frequently in the document set and increases the weight of terms that occur rarely.
Karen Spärck Jones (1972) conceived a statistical interpretation of term specificity called Inverse Document Frequency (idf), which became a cornerstone of term weighting:
- The specificity of a term can be quantified as an inverse function of the number of documents in which it occurs.[4]
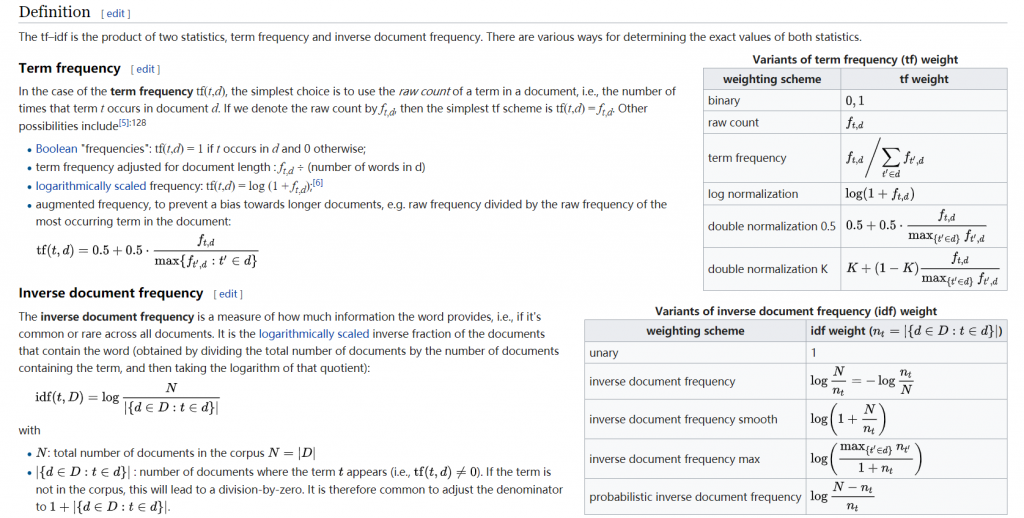
Term frequency–Inverse document frequency[edit]
Then tf–idf is calculated
A high weight in tf–idf is reached by a high term frequency (in the given document) and a low document frequency of the term in the whole collection of documents; the weights hence tend to filter out common terms. Since the ratio inside the idf’s log function is always greater than or equal to 1, the value of idf (and tf–idf) is greater than or equal to 0. As a term appears in more documents, the ratio inside the logarithm approaches 1, bringing the idf and tf–idf closer to 0.
注意:tf-idf= tf x idf 。idf是为了避免无意义的单词干扰预测结果,比如,英文中的”the“这种单词在句子中经常出现, 所以它的tf可能会很高,但是”the“的对于判定相关性并无太大意义,所以需要用 idf来减少其权重,而对于那些很少出现但对相关性有帮助的词,增加其权重。
tf-idf的加权方法有下面常见的几种:
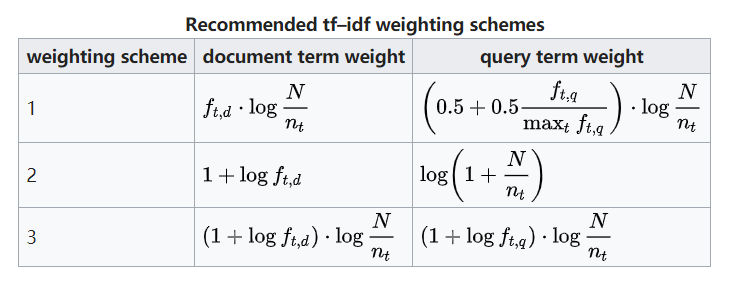
垃圾短信检测:采用监督学习的方式,计算每条短信的词根的tf-idf值,形成特征向量, 然后 使用Naive Bayes classifier 用打上了标签的数据进行训练, 在用分类器对新的短信进行分类。 不过分词和词库很关键。
来源:https://en.wikipedia.org/wiki/Tf%E2%80%93idf